Artificial Intelligence
AI Predicts Alzheimer's Disease Risk Seven Years in Advance
AI enables precision medicine with early detection of Alzheimer's disease risk.
Posted February 25, 2024 Reviewed by Tyler Woods
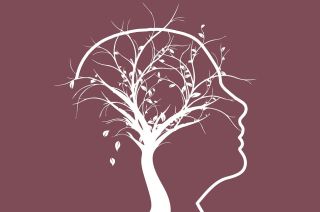
Researchers at the University of California, San Francisco (UCSF) recently developed an AI algorithm that can identify patients at risk for developing Alzheimer’s disease up to seven years in advance, according to a study published last week in Nature Aging.
The researchers reported that their AI models predicted Alzheimer’s disease up to seven years in advance with 72% accuracy.
The predictive capabilities of artificial intelligence (AI) machine learning are enabling disease prediction and accelerating precision medicine in an effort to improve patient outcomes. Alzheimer's disease (AD), an incurable neurodegenerative disease, is the most common form of dementia.
Alzheimer's disease progressively affects a person's memory, cognition, and ability to perform daily tasks, according to the National Institute on Aging. The disease is named after neurologist Alois Alzheimer, who, in 1906, found tangled fiber bundles and abnormal clumps in the brain of a woman during a postmortem examination. Today, those fibers are known as neurofibrillary or tau tangles, and the abnormal clumps are known as amyloid beta (proteins Aβ), and both are among the main hallmarks of Alzheimer’s disease.
“Identification of Alzheimer’s disease (AD) onset risk can facilitate interventions before irreversible disease progression,” wrote UCSF study lead author Alice Tang, a researcher at Sirota lab, the laboratory of senior author and associate professor Marina Sirota, Ph.D., along with their research colleagues.
Lifestyle changes may prevent up to one-third of Alzheimer's disease cases according to WAM, thus having a way to predict those at high-risk years in advance is important.
Currently, there are an estimated 24 million people with Alzheimer’s. The disease impacts nearly one-third of those over age 85 and one in ten over 65 years old around the world, according to the Cleveland Clinic. Dementia cases are predicted to increase significantly worldwide. By 2050, roughly over 150 million people globally will have Alzheimer's disease, according to a different study published in The Lancet Public Health.
In the U.S., someone develops Alzheimer’s disease every 65 seconds, according to the Women’s Alzheimer’s Movement (WAM), founded by Maria Shriver in partnership with the Alzheimer’s Association, which is now part of the Cleveland Clinic. Among the 6.7 million Americans living with Alzheimer's, two-thirds are women, according to WAM. Researchers have yet to scientifically prove exactly why a majority of U.S. Alzheimer’s cases are women.
The UCSF researchers used a precision medicine database tool called SPOKE (Scalable Precision Medicine Knowledge Engine) that was developed in the lab of UCSF neurology professor Sergio Barazini, Ph.D., in collaboration with Sharat Israni, Ph.D., the executive director of UCSF’s Bakar Computational Health Sciences Institute. SPOKE is a growing network, currently consisting of over 25 databases, that enables biomedical researchers to understand the interconnected pathways of disease, epigenomics, clinical phenotypes, genes, proteins, drug compounds, tissues, organs, environment, lifestyle, and more data.
Artificial intelligence machine learning algorithms require massive amounts of training data in order to figure out the relevant features from the data in order to perform tasks such as predictions without hardcoding explicit commands via computer programming.
The UCSF researchers attribute the lack of understanding of how Alzheimer's disease develops, the pathogenesis, partially due to the paucity of data that connects clinical and molecular realms and the lack of longitudinal data, or data on the same patients over long periods of time.
The UCSF team set out to develop AI models based on clinical data from electronic health records that can predict Alzheimer's disease and used the SPOKE network to help understand the possible biological reasons for the identified predictors. From over 5.5 million electronic health records from 1980-2021, the data used for the study include nearly 3,000 patients diagnosed with Alzheimer's by the UCSF Memory and Aging Center.
The team used binary classification AI models, as well as random forest algorithms, which are widely used AI machine learning algorithms that use the combination of the result of many decision trees to yield a single result. Random forest algorithms are flexible, less likely to have the problem of overfitting, and make it easy to spot the importance of features.
High cholesterol (hyperlipidemia or HLD) was a predictor for Alzheimer’s across all AI models, with the APOE (apolipoprotein E) gene as a shared causal genetic link in this study. This is in line, and validates, other studies that have shown that the APOE4 gene is the most common late-onset (occurring after 65 years of age) Alzheimer's gene. The researchers found that the IL6, INS, and ACTB genes were top predictors of AD as well.
In males, an enlarged prostate (prostatic hyperplasia) is predictive of AD. Interestingly, the AI models and SPOKE revealed a link for females between the MS4A6A gene and osteoporosis as a predictor for Alzheimer’s disease, which was not the case for males.
“These findings of osteoporosis as a potential sex-specific predictor of AD, with shared relationships through MS4A6A, is an unknown and unexpected result identified from single-hypothesis-driven follow-up from our prediction models,” the UCSF researchers reported. Additional studies would need to be conducted to validate the underlying reasons why.
The combination of AI machine learning, molecular databases, electronic health records, and the precision medicine database search tool SPOKE enables researchers to identify those at high risk for developing Alzheimer’s disease, understand why, and facilitate precision medicine in hopes of better patient outcomes in the future.
References
Copyright © 2024 Cami Rosso All rights reserved.