Bias
Can Statistics Ever Be Biased?
The truth behind “lies, damned lies, and statistics.”
Posted November 14, 2023 Reviewed by Abigail Fagan
Key points
- There’s a difference between statistics and cases of misstating, misusing, or lying about statistics.
- Statistics and science that disprove our personal beliefs are especially likely to be unfairly criticized.
- There are ways to train the populace in statistical literacy to not fall for the misuses of statistics.
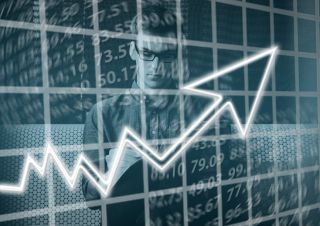
Statistics and science have been publicly slammed by some. The issues of climate change and COVID-19, in particular, have stirred accusations of bias, conspiracies, and even hoaxes (Philipp-Muller et al., 2022). But can the statistics themselves ever really be biased? As a researcher and statistics instructor, I think this issue is pretty straightforward. The answer is no.
Statistics Versus Lies
There is that famous quote of “three kinds of lies: lies, damned lies, and statistics.” Some books critical of social science have drawn from this idea, including Joel Best’s two editions of “Damned Lies and Statistics: Untangling Numbers from the Media, Politicians, and Activists.”
But Best’s (2012) best example of a biased statistic, or what he called “the most inaccurate social statistic ever,” was actually nothing more than a misquote. Best investigated a result that, as of 1994, “every year [italics added] since 1950, the number of American children gunned down has doubled.” Best criticized it because the actual result was that “the number of American children killed each year by guns has doubled since 1950.” If 1,000 children were killed by guns in 1950, then the latter quote indicates about 2,000 were killed in 1994, whereas the former misquote doubling the number from year to year would indicate trillions of such deaths after the 44 years.
Best bemoaned the sloppy paraphrasing of the original result and the lack of verification from editors. But the doubling-every-year result was not even the actual statistic. It was a lie or at least an untruth. And none of that confusion was the fault of statistics. It was human bias or error.
Statistics can be defined as the summary or analysis of numerical data. Statistics are factual. They are dry, objective outcomes of applying mathematical tools to a set of data. They are nonetheless often criticized for other reasons.
Made Up or Misquoted
Occasionally, there are cases where scientists fabricate their results. These individuals deserve sanction but not the whole field, similar to disbarring a lawyer who violates a code of ethics or arresting a CFO who doctors the books—it’s not the general practice of law or business at fault.
Misstating or miscalculating a result due to a lack of care or a subconscious bias is less nefarious but still concerning. I have uncovered several such instances. Contrary to common reporting, most participants saw the “invisible gorilla” (Stalder, 2018), victims are generally more likely to receive help the more bystanders there are (Stalder, 2008), and socially smart people are more prone to some interpersonal biases (Stalder, 2014). But I don’t attribute the mistakes to statistics that lie.
Misinterpreted
Similarly, there can be misinterpretations. There are numerous cases of correlational studies being misreported in cause-effect terms (Stalder, 2021). Correlation does not imply causation. Some who infer causation from correlation even assert that “the statistics don’t lie,” as when a civil rights group accused a Michigan police department of racially profiling motorists (Samra, 2023).
In racial profiling, the race of motorists causes police to target them, but with only correlational evidence, the cause for disproportionate attention to Black motorists is technically unverified (Kowalski and Lundman, 2007). Yes, the statistics are valid, and disproportionate treatment is concerning. But a cause-effect interpretation of such a result may still be premature. In another interview, leaders of the same civil rights group acknowledged that “they don’t know [the cause] with certainty” (Neavling, 2023).
Misused to Mislead
Sometimes a valid statement of a result can be misleading. It turns out, for example, that there are many more poor White people in the United States than poor Black people. That fact should not be ignored in understanding poverty and race, but it’s based on frequency data, whereas it’s also true and less misleading to state that the proportion of Whites who are poor is much smaller than for Blacks. It’s just that there are so many more Whites in this country in the first place.
Similarly, using frequency data, Whites are more likely to be both victims of violent crime and perpetrators of it (Best, 2012)—a politician can choose whichever supports their view. Political advantage has also been gained by manipulating the axes of a graph, to show, for example, that COVID-19 cases or world temperatures were going down when they were actually not or doing the opposite (Calzon, 2023).
Capable of Disproving Beliefs
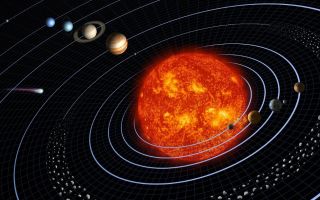
There are many examples of statistics and science disproving our strongly held beliefs, including the once-very-common belief that the earth was at the center of the universe. In general, we can get defensive or upset when science contradicts our views. As predicted by cognitive dissonance theory, it is rare to change our mind right away. It is much more common to attack the messenger, especially if we belong to an already anti-science group (Philipp-Muller et al., 2022). The “damned” part in the “damned lies” accusation can possibly reflect our emotional involvement when our personal views are contradicted, not that science is never wrong.
Acknowledgment
In fairness, some statistical tests are known to be less mathematically conservative than others, in that it’s easier to declare a “statistically significant” result. So in a manner of speaking, perhaps it’s fair to call a less conservative test a biased statistic, although trained reviewers can take this issue into account before accepting a research-based article for publication.
In Sum
Those who use or communicate statistics, including scientists, broadcasters, bloggers, and politicians, can be biased and even deceitful. Don’t trust every supposedly science-based conclusion on its face. The results reported and replicated in peer-reviewed journals are more likely to be valid. But even if someone misquotes, misinterprets, or misleads, it’s not something inherently deceitful or faulty in the statistics. There are ways to train the populace in statistical literacy to understand this distinction (The Open Minds Foundation, 2023). Despite these challenges, the world needs statistics to help address its problems (Best, 2012).
References
Joel Best, Damned Lies and Statistics: Untangling Numbers from the Media, Politicians, and Activists, 1st edition, updated (Berkeley, CA: University of California Press, 2012).
Bernardita Calzon, “Misleading Statistics Examples—Discover the Potential for Misuse of Statistics and Data in the Digital Age,” datapine (blog), January 6, 2023, https://www.datapine.com/blog/misleading-statistics-and-data/#:~:text=Misleading%20statistics%20refers%20to%20the,news%2C%20media%2C%20and%20others.
Brian R. Kowalski and Richard J. Lundman, “Vehicle Stops by Police for Driving While Black: Common Problems and Some Tentative Solutions,” Journal of Criminal Justice 35 (2007): 165–81.
Steve Neavling, “Alarming Racial Disparity Found in Ferndale Arrests and Traffic Tickets—Again,” Detroit Metro Times, August 17, 2023.
The Open Minds Foundation, “Relearning Statistics Can Reduce Your Risk of Manipulation,” Psychology Today, October 31, 2023, https://www.psychologytoday.com/us/blog/the-art-of-critical-thinking/202310/relearning-statistics-can-reduce-your-risk-of-manipulation.
Aviva Philipp-Muller et al., “Why Are People Antiscience, and What Can We Do About It?,” PNAS 119 (July 12, 2022).
Ibrahim Samra, “Report Reveals Large Racial Disparity Among Ferndale Tickets, Arrests Involving Black Drivers,” CBS News, August 17, 2023, https://www.cbsnews.com/detroit/news/report-reveals-large-racial-disparity-among-ferndale-tickets-and-arrests-involving-black-drivers/.
Daniel R. Stalder, “Are Attributionally Complex Individuals More Prone to Attributional Bias?” (presentation, Annual Convention of the Midwestern Psychological Association, Chicago, IL, May 1–3, 2014).
Daniel R. Stalder, “False Cause Fallacy Reaches the Olympics,” Psychology Today, September 21, 2021, https://www.psychologytoday.com/us/blog/bias-fundamentals/202109/false-cause-fallacy-reaches-the-olympics.
Daniel R. Stalder, The Power of Context: How to Manage Our Bias and Improve Our Understanding of Others (Amherst, NY: Prometheus Books, 2018).
Daniel R. Stalder, “Revisiting the Issue of Safety in Numbers: The Likelihood of Receiving Help from a Group,” Social Influence 3 (2008): 24–33.