Artificial Intelligence
AI Helps Explain What the Brain Finds Memorable
Researchers use AI to help unravel neuroscience mystery.
Posted May 30, 2024 Reviewed by Gary Drevitch
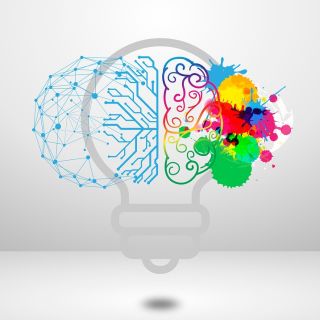
Why does the brain remember some images and not others? One of the fundamental questions of neuroscience is how perception impacts what we remember. Yale University researchers have published a new peer-reviewed neuroscience study in Nature Human Behaviour that uses artificial intelligence (AI) and behavioral science to help explain why some images make a lasting impact while others are filtered out by the brain.
The Yale researchers created a computational model to mimic in silico the memory-forming steps of the compression and reconstruction of visual signals. According to the scientists, their study illustrates the usefulness of model-driven psychophysics, the branch of psychology that studies the relationship between physical processes such as stimuli on the mental process of an organism.
“Much of what we remember is not because of intentional selection, but simply a by-product of perceiving,” wrote senior authors Ilker Yildirim, an Assistant Professor of Psychology and Lab Director at the Cognitive & Neural Computation Laboratory, and John Lafferty, an Associate Director of the Wu Tsai Institute, Director of the Center for Neurocomputation and Machine Intelligence, and John C. Malone Professor of Statistics and Data Science, along with co-first authors former Yale graduate students Qi Lin and Zifan Li. “This raises a foundational question about the architecture of the mind: How does perception interface with and influence memory?”
The Yale researchers created a sparse coding model (SPC) that was trained to reconstruct the activations in a given layer of an AI deep convolutional neural network (DCNN) trained for image classification. Sparse coding is an AI machine learning method that is often used for brain imaging, sound processing, image processing, and natural language processing. Sparse coding enables an efficient, more compact representation of complex data. For this study, the sparse coding model consists of an input layer, an intermediate layer for recoding the inputs, and a reconstruction layer for reproducing the input. Their sparse coding model was trained to reconstruct the AI deep convolutional neural network activations conjured by over 10,200 images of nature scenes with the goal of minimizing reconstruction errors. The reconstruction error was a predictor of not only memory accuracy, but also response times.
“We observe that the hard-to-reconstruct images tend to be more object-centered or contain humans, relative to the easy-to-reconstruct images,” the Yale researchers noted.
Next, the researchers had 45 study participants view natural scenes in a rapid stream and then tested them for what images they remembered. The images that the participants remembered the most were the ones that were more difficult for their sparse coding model to reconstruct. In short, images with large reconstruction errors were found to be more memorable.
“This work establishes reconstruction error as an important signal interfacing perception and memory, possibly through adaptive modulation of perceptual processing,” the scientists concluded.
Copyright © 2024 Cami Rosso All rights reserved.