Artificial Intelligence
AI Performance Enhanced With Human Developmental Psychology
A new model applies childhood learning concepts to achieve greater accuracy.
Posted June 13, 2024 Reviewed by Gary Drevitch
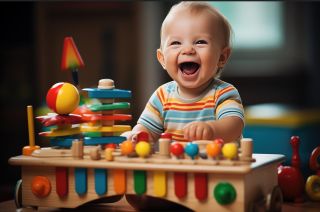
Researchers at The Pennsylvania State University (Penn State) published a new study that shows how a novel artificial intelligence (AI) machine-learning method using concepts from human developmental psychology and childhood learning achieve nearly 15% greater accuracy.
“The field of developmental psychology offers insights into what is missing from contemporary machine vision learning,” wrote Brad Wyble, corresponding author and psychology professor, James Z. Wang, distinguished professor of information sciences and technology, and researchers Wonseuk Lee and Lizhen Zhu at Penn State.
The performance accuracy of AI deep learning often requires vast amounts of training data. Even with exposure to gargantuan amounts of training data, today’s AI machine learning lacks the ability to generalize, a requisite for artificial general intelligence. For example, the large language model (LLM) chatbot ChatGPT was trained from massive amounts of data from the internet, licensed third-party data, and information from users and human trainers, according to OpenAI.
“Despite being trained on extensive datasets, current computer vision systems lag behind human children in learning about the visual world,” the researchers wrote.
Humans are naturally able to generalize concepts when presented with entirely new material. It is often posited by the tech-savvy that young children are more intelligent than the most sophisticated AI system. For example, a child can learn the concept of a cat and correctly identify other cats with limited exposure without having to be explicitly exposed to or taught every feline species prior.
By the time a child is two years old, they have made roughly 90 million visual fixations from their environment, an amount that is much less than the massive AI-training datasets containing up to billions of images, according to the researchers. The visual fixations consist mostly of a limited set of faces, objects, and perspectives in their household.
“While viewing the world, children harness a wealth of environmental information about how their bodies deliberately sample information through controlled orientation of their senses and their interactions with the world,” the scientists wrote.
Can mimicking how a human child learns in silico lead to overall smarter, better-performing AI algorithms? The scientists posit that human location perception is key to infant visual learning and reasoned that incorporating environmental context to AI machine learning will enable learning that will be flexible enough to perform better on tasks such as classification using less data.
Drawing upon human developmental psychology, the researchers decided to use the environment as a contextual data source, an approach they named environmental spatial similarity (ESS). The team created a simulation of an agent moving through a furnished house and apartment using a platform for interactive physical simulations called ThreeDWorld (TDW) and state-of-the-art ray tracing to create realistic shadows, reflections, and material properties.
The simulation had realistic features such as glossiness that “that mimic the appearance of real gloss in human psychophysics.” Psychophysics is the field of psychology that studies the relationship between the physical aspects of a stimulus and the reported sensations and perceptions—the relationship between the material and mental. The term was coined by physicist, professor, philosopher, and experimental psychologist Gustav Theodor Fechner (1801-1887).
For this study, the Penn State researchers modified a self-supervised AI learning algorithm called the momentum contrast (MoCo) that was created in a different study published in 2020 by researchers at Meta FAIR labs (formerly Facebook AI Research) and implemented it using Pytorch.
The Penn State algorithm takes into account contextual spatial data from simulation images in order to identify image pairs that are close in spatial and rotational coordinates and to allow for multiple pairs. They compared their AI model’s performance in a variety of conditions to the base model MoCo (version 2) algorithm.
The new ESS approach outperformed the baseline model on a variety of tasks, including up to 15% (14.99%) better with a 99.35% average accuracy in the task of identifying the room in a virtual apartment.
According to the researchers, there are a number of real-world applications that their new AI approach can benefit, such as human vision, neuroscience, computer vision, disaster relief, autonomous aircraft, robotics, and even planetary and space exploration in the future.
Copyright © 2024 Cami Rosso All rights reserved.