Artificial Intelligence
Should We Use AI to Personalize Our Options?
It sounds good in theory, but may backfire in practice.
Posted January 8, 2024 Reviewed by Devon Frye
Key points
- AI has the potential to offer us personalized options.
- But too much personalization may come with downsides and actually detract from our decision-making.
- AI personalization may also allow third-parties to subtly influence our choices.
- Though AI can offer ways to improve our decisions, there will always be trade-offs.
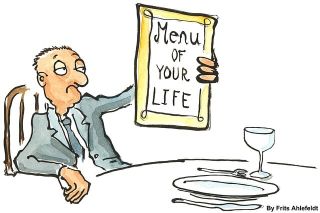
I recently came across an article from Schneier (2023) about the potential for AI to remove “bottlenecks between what you want and what you get.” The central idea is that AI has the potential to better connect what you want with the available options, so that you are choosing from a more personalized list of choices.
For example, if you go to a restaurant, you’re currently limited to the options available on the menu. But AI has the potential to connect your food preferences with the capabilities of that restaurant to offer you a personalized list of options. Such a tool could lead to unimaginable personalization of one’s life, from less important aspects of life, such as food and clothing, to much more impactful aspects of life, such as jobs and voting.
So, that got me to thinking: Would the ability to have fully tailored options produce a net benefit in one’s life? Though intuitively the answer is yes, there are some issues to consider that may add nuance to that answer.
Knowing What You Want
We are notoriously bad at predicting what we want. For example, Ansari (n.d.) noted that there’s often a clear disconnect between what people claim they want in a romantic partner and what they actually want. People think they want a partner who possesses a list of traits, but it turns out there’s a hierarchy at play—for most people, it begins with physical attractiveness and goes from there.
In his book Stumbling on Happiness, [1] Dan Gilbert (2006) argues that we’re just generally not very good at predicting what will make us happy. Although he delves into some of the reasons why, the central idea is that happiness is an abstract concept and not one where there’s a clear, concrete, and obvious way to obtain it.
Hence, while AI could use data about prior decisions to help personalize options for us, the use of said data is likely to be limited to relatively simple decisions, such as food or clothing choices. But as we move to more abstract decisions, such as those involving who to date or deciding a nuanced stance on a political issue, personalizing options using AI would require much more input from the user. And since we often struggle to articulate abstract ideas in a precise, concrete way, there’s a greater likelihood of error when it comes to personalized options.
In addition, as I've written about previously, the values we practice are situationally contingent, meaning we don’t practice our values equally across situations. As such, determining where we fall on a given value or preference in an absolute sense is extremely difficult to untangle.
You might say that you want a romantic partner who possesses traits X, Y, and Z (e.g., caring, funny, ambitious). What are the constraints around those traits? For example, even if you could easily specify what you find funny (a hard task, I suspect), in which situations would you want that? Closing the gap between what you say and what you mean would be quite the challenge (also called alignment, a problem we have yet to resolve; Mitchell, 2022) [2].
So, for simple decisions, it might be easy for the AI to offer us personalized options, but as we move to more complex decisions that involve more abstract concepts, there’s an increased potential for alignment problems. But that’s not the only issue of relevance.
The Paradox of Choice
Imagine a restaurant that offers a menu with several attractive options. You might struggle to know exactly what to order because of two or three dishes that sound good. You might even wrestle with the decision [3].
But imagine if everything on the menu was something that sounded good. The volume of choices may become overwhelming.
This phenomenon is referred to as the paradox of choice—we tend to have an easier time making decisions when there are fewer viable options to choose from. This doesn’t necessarily mean the fewer choices the better (Decision Lab, n.d.). It means that as the number of viable options increases, decision difficulty also tends to increase.
If instead of choosing from a list of items on the standardized menu where two or three choices sound good, imagine how much more difficult it would be if you had a larger personalized list of choices (an example used by Schneier) [4]. We would run the risk of having too many attractive options to choose from, potentially leading to decision paralysis and detracting from the overall experience.
The Age of Average
Most decisions we make involve us choosing from a list of options that includes some unsatisfactory choices. Imagine the last time you went shopping for something online. I suspect your search produced many options that you deemed to be unsatisfactory.
AI, though, offers the potential for the list of options to include only those predicted by your preferences, so you don’t have to waste time reviewing unsatisfactory options [5]. Imagine telling your AI assistant what you’re looking for, and it presents you with a variety of options that are predicted to meet your needs. That seems like a much more efficient way to go about things, at least in theory.
For the AI to be able to personalize your list of options, it will use data on your past decisions. Beyond the data privacy issues that would have to be addressed for the AI to be able to personalize your options with any reasonable accuracy [6], there’s a potential unintended consequence for your decision-making.
Though not specific to AI, Alex Murrell (2023) wrote an article called “The Age of Average.” In it, he details how increased access to data has led to an overwhelming level of sameness among choices (e.g., book covers, images, storylines). Because personalization is based on prior decision data, there’s the potential for those options to be limited to those predicted to be viable based on past decision history.
This could eliminate options that would be novel based on your past decision history. So, if you want to try something new (e.g., a new genre of book, a new type of food), your AI assistant may be useless to you.
And the more you rely on the AI to aid you in decision-making, the more limited your choices may become over time. For example, consider the use of an app to order a pizza from your favorite place. On the app, you have a list of toppings, but (a) there are one or two you almost always include, (b) there are a few you occasionally include, and (c) there are some you never include.
Over time, the AI is likely to begin limiting your list of topping options because it’s programmed to use your past data to predict a personalized list. So, the first items to go will be those you never choose. That might not be much of a problem, but if you also tend to have a strong bias toward some choices, eventually those choices may become the only ones you’re given.
Potential Third-Party Influence
A while back I wrote about choice architecture, which concerns the deliberate attempt to present choices in a way that influences the resulting decision. One of the issues I brought up relates to the potential that more than just user preferences will determine your personalized list of options.
So, if you want to buy a book to read, the AI will use data on your reading preferences (and reading history) to offer you a selection of choices that should meet your needs. But there’s also the potential that other data could be used.
For example, the AI could rely on third-party data (e.g., publishers, activist groups, politicians, online stores) to influence your list of personalized options [7]. In the end, your personalized list might also be influenced as much by third-party preferences as your own.
There Are Trade-Offs Galore
An underlying assumption apparent in the Schneier article (and others like it, such as Josifovsk, 2023, and Clark, 2021) is that the use of AI to increase personalization leads to users getting exactly what they want. But as I’ve argued here, the increase in AI-driven personalization may increase the risk of:
- Making our decision-making more difficult (due to difficulty choosing among options).
- Decreasing novelty in our decision choices.
- Giving third parties greater, but less obvious, influence over our decisions.
None of these are guaranteed to occur, of course. As AI becomes more sophisticated, there are going to be many opportunities where it can be used to improve human decision-making.
But it’s important to recognize there will likely be trade-offs when it comes to the benefits of more personalized options. Whether those trade-offs will be worth it and whether increased personalization will introduce unintended consequences remains to be seen.
References
Footnotes
[1] It’s an exceptional read if you’re interested. If you want more of a quick hit on Gilbert, check out a TedTalk he gave in 2004 or a good summary blog by Zuckerman (2009) based on a sit-down interview with Dan.
[2] There is an expression for this, called “Do what I mean,” which can be abbreviated as DWIM.
[3] You’ll eventually choose something, though, or else you won’t eat.
[4] He also includes the example of the restaurant having already started on a “meal optimized for your tastes,” but there are likely even more challenges to effectively doing that.
[5] You must put aside the current deficiencies in many of the current AI-driven recommendations we currently receive. Here, we are assuming such recommendations have gotten much more valid.
[6] This is not meant to minimize the importance of those issues, but I am assuming here such issues have been addressed.
[7] This is like how a site’s recommendations currently work. For example, Amazon isn’t going to recommend products not sold on Amazon, even if those products might be a better fit for your needs.