Artificial Intelligence
AI Enables Virtual Behavioral Neuroscience
Realistic AI rodents may accelerate psychobiology, robotics, biotech, and pharma.
Posted June 14, 2024 Reviewed by Ray Parker
Key points
- AI can be used to create realistic virtual animals.
- The virtual rodents can help us understand how real ones move.
- This new technique could accelerate progress in both artificial intelligence and neuroscience.
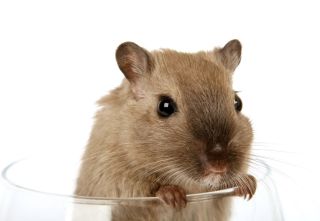
Understanding the underlying biological mechanisms of human and animal behavior can help advance critically important industries such as medicine, healthcare, robotics, artificial intelligence (AI), and more. On Tuesday, scientists at Harvard University and Google DeepMind published a new study in Nature that shows how AI deep reinforcement learning can be used to create a realistic virtual rodent that may help advance behavioral neuroscience and vital research across many fields.
Behavioral neuroscience, also known as psychobiology, physiological psychology, biopsychology, or biological psychology, is the study of the neural and biological basis underlying behavior in humans and animals. It is an interdisciplinary discipline that combines elements from physics, biology, chemistry, mathematics, and psychology. Psychobiology is useful for robotics, artificial intelligence, developmental psychology, cognitive psychology, psychiatry, neuroendocrinology, audiology, biochemistry, drug discovery, biotechnology, health care, medicine, assistive technology, pharmaceutical, speech-language pathology, veterinary, and other fields.
“Animals have exquisite control of their bodies, allowing them to perform a diverse range of behaviors,” wrote Bence P. Ölveczky, Ph.D., a professor of organismic and evolutionary biology for brain science at Harvard, who led the study in collaboration with Josh Merel, Jesse D. Marshall, Leonard Hasenclever, Ugne Klibaite, Amanda Gellis, Yuval Tassa, Greg Wayne, Diego Aldarondo, and Matthew Botvinick. “How such control is implemented by the brain, however, remains unclear.”
How does one go about creating a realistic virtual mammal in silico—in this case, algorithmically modeling rats in motion? For this study, the AI design includes a vision encoder, a proprioceptive encoder, a core module trained by backpropagation, and a policy module consisting of one or more long short-term memory (LSTM) recurrent neural networks.
The researchers wrote,
“We used deep reinforcement learning to train the virtual agent to imitate the behavior of freely moving rats, thus allowing us to compare neural activity recorded in real rats to the network activity of a virtual rodent mimicking their behavior.”
Deep reinforcement learning is a type of AI machine learning that combines deep neural networks with reinforcement learning in a manner that allows an agent to learn behavior through the results of actions. Deep neural networks (DNN) are a type of artificial neural network (ANN) with an input layer, an output layer, and many hidden layers for processing and passing data in between. The greater the number of layers, the deeper the neural network.
In artificial intelligence, reinforcement learning algorithms learn from outcomes to determine the next steps with the goal of achieving the best outcome to maximize the reward. Reinforcement learning (RL) is a type of AI machine learning that simulates learning by trial and error and feedback refined by environmental interaction. Reinforcement learning is used for complex, real-world scenarios where present-day decisions impact future outcomes. The type of reinforcement can be positive, negative, punishment, extinction, intermittent, or continuous. A real-world example of a type of analog reinforcement learning is giving a pet dog a food treat when commanded to “sit” as a reward and form of positive reinforcement. Reinforcement learning is used for robotics, autonomous vehicles, healthcare, computer gaming, recommendation engines, personalized medicine, and more uses.
The researchers developed a virtual rat body using a physics-based engine for model-based control and actual measurements of laboratory rats. They then tasked the virtual rodent with a variety of tasks, such as jumping, foraging, escaping, and double touching. Scientists reported,
“We found that neural activity in the sensorimotor striatum and motor cortex was better predicted by the virtual rodent’s network activity than by any features of the real rat’s movements, consistent with both regions implementing inverse dynamics.”
This is an exciting development that may accelerate both artificial intelligence and neuroscience. According to the scientists, they can completely monitor neural activity, behavior, and sensory inputs, as well as the model’s training goals, variance sources, and connectivity.
“These results demonstrate how physical simulation of biomechanically realistic virtual animals can help interpret the structure of neural activity across behavior and relate it to theoretical principles of motor control,” concluded the researchers.
Copyright © 2024 Cami Rosso. All rights reserved.